Role of AI and Machine Learning in Drug Discovery
Drug Design and Optimization: AI can analyze large datasets of molecular structures, properties, and interactions to predict which compounds are most likely to be effective as drugs. This speeds up the process of identifying potential drug candidates and optimizing their properties.1
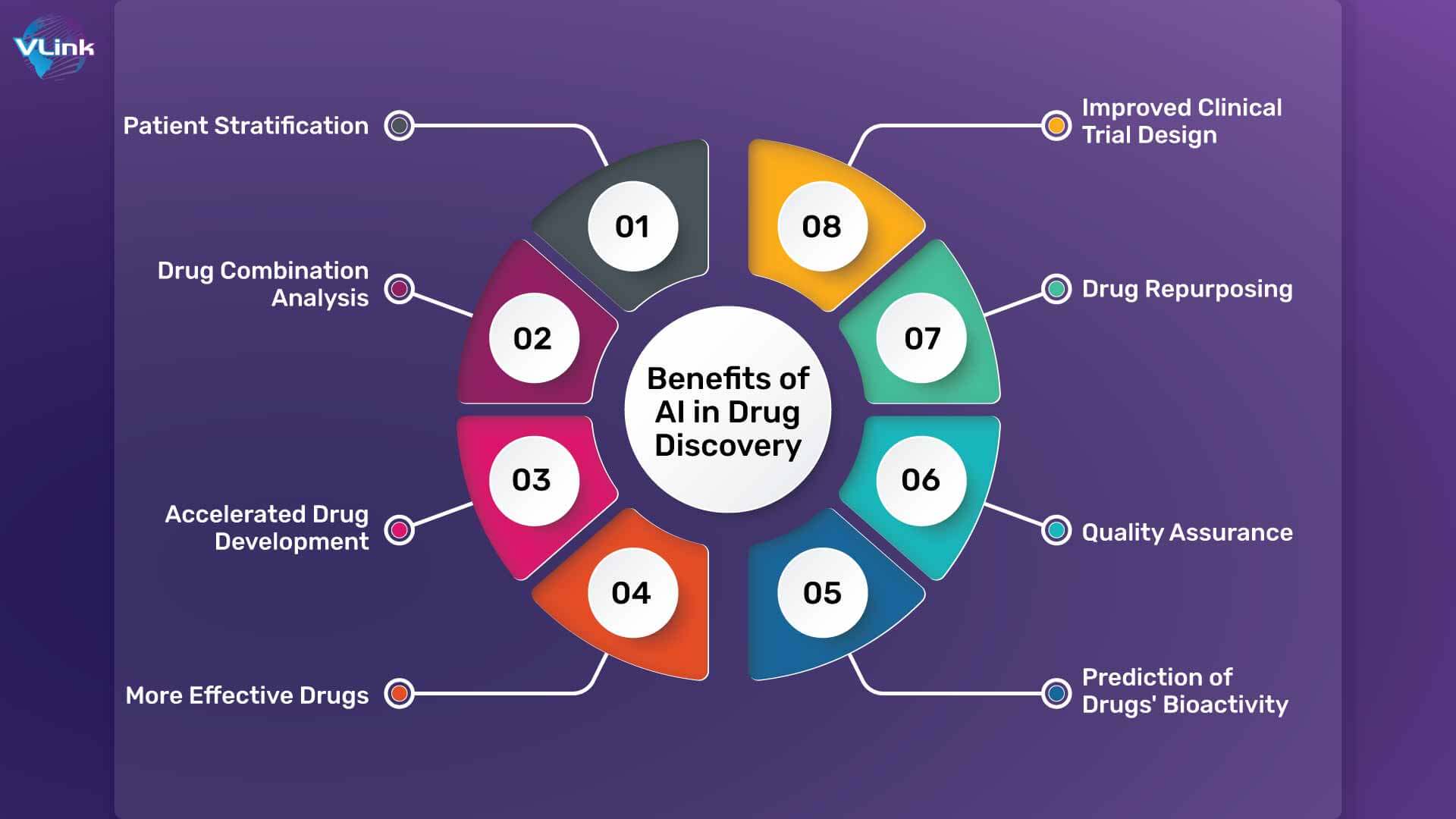
Target Identification: Machine learning algorithms can sift through biological data to identify potential targets for drug intervention. By analyzing genetic, proteomic, and metabolic data, AI can pinpoint biomarkers or molecular pathways that play key roles in diseases.
Predicting Drug-Target Interactions: AI models can predict how drugs interact with specific biological targets, which helps researchers understand potential efficacy and side effects early in the drug development process.
Virtual Screening: Instead of testing thousands of compounds in the lab, AI algorithms can perform virtual screening of vast libraries of compounds. This reduces the number of experiments needed by suggesting the most promising candidates for testing.
Drug Repurposing: AI can analyze existing drugs and their known effects to suggest new therapeutic uses. By identifying unexpected interactions or off-target effects, AI helps researchers find new indications for existing drugs faster and more efficiently.
Clinical Trial Optimization: Machine learning can analyze patient data to identify suitable candidates for clinical trials and predict which patients are likely to respond best to a new treatment. This helps streamline the trial process and improve success rates.
Personalized Medicine: AI can analyze large-scale patient data, including genetic information and medical histories, to tailor treatments to individual patients. This approach enhances treatment efficacy and reduces adverse effects by matching therapies to patient-specific characteristics.
Drug Safety: AI can predict potential side effects or safety concerns by analyzing chemical structures and known biological effects. This proactive approach helps identify safety issues early in the development process.
Data Integration and Decision Support: AI tools can integrate diverse sources of data from genomics, proteomics, clinical records, and scientific literature to provide comprehensive insights and support decision-making throughout the drug discovery pipeline.
Generative Chemistry: AI algorithms can generate novel chemical structures that meet specified criteria, such as target affinity and pharmacokinetic properties. This capability expands the range of chemical space explored for potential drug candidates.
Biological Image Analysis: Machine learning techniques can analyze complex biological images, such as those from high-content screening assays or medical imaging data, to extract meaningful information about disease mechanisms or drug effects.
Natural Language Processing (NLP) for Drug Literature: AI-powered NLP can extract valuable insights from vast amounts of scientific literature, including research papers, patents, and clinical trial reports. This helps researchers stay updated with the latest findings and discoveries.
Drug Dosage Optimization: AI algorithms can optimize drug dosing regimens based on patient-specific factors, such as age, weight, genetics, and disease progression. This personalized approach improves treatment efficacy and patient outcomes.
Drug Manufacturing and Quality Control: AI-driven processes can optimize drug manufacturing processes to improve efficiency, reduce costs, and ensure consistent quality. Machine learning models can also predict and prevent manufacturing defects.
Drug Combination Therapy: AI can analyze data on drug interactions and synergies to identify effective combinations for treating complex diseases, such as cancer or infectious diseases. This approach leverages the complementary effects of different drugs to enhance therapeutic outcomes.
Real-time Data Analysis: AI enables real-time analysis of streaming healthcare data, such as patient vital signs or electronic health records, to identify patterns indicative of disease progression or treatment response. This capability supports adaptive clinical decision-making.
Ethical and Regulatory Compliance: AI tools can assist in navigating complex regulatory landscapes by analyzing regulatory guidelines, identifying potential compliance issues, and ensuring that drug development processes adhere to ethical standards and regulations.
Drug Pricing and Market Access: AI can analyze market data, healthcare economics, and patient outcomes to optimize drug pricing strategies and improve market access. This helps ensure that new therapies are affordable and accessible to patients who need them.
Continual Learning and Adaptation: AI systems can learn from ongoing clinical data and patient feedback to continuously refine drug development strategies and improve treatment protocols. This iterative process supports ongoing innovation and adaptation in healthcare.
Global Collaboration and Knowledge Sharing: AI facilitates collaboration among researchers, clinicians, and pharmaceutical companies across geographical boundaries. By sharing data, insights, and methodologies, AI accelerates collective progress in drug discovery and development..